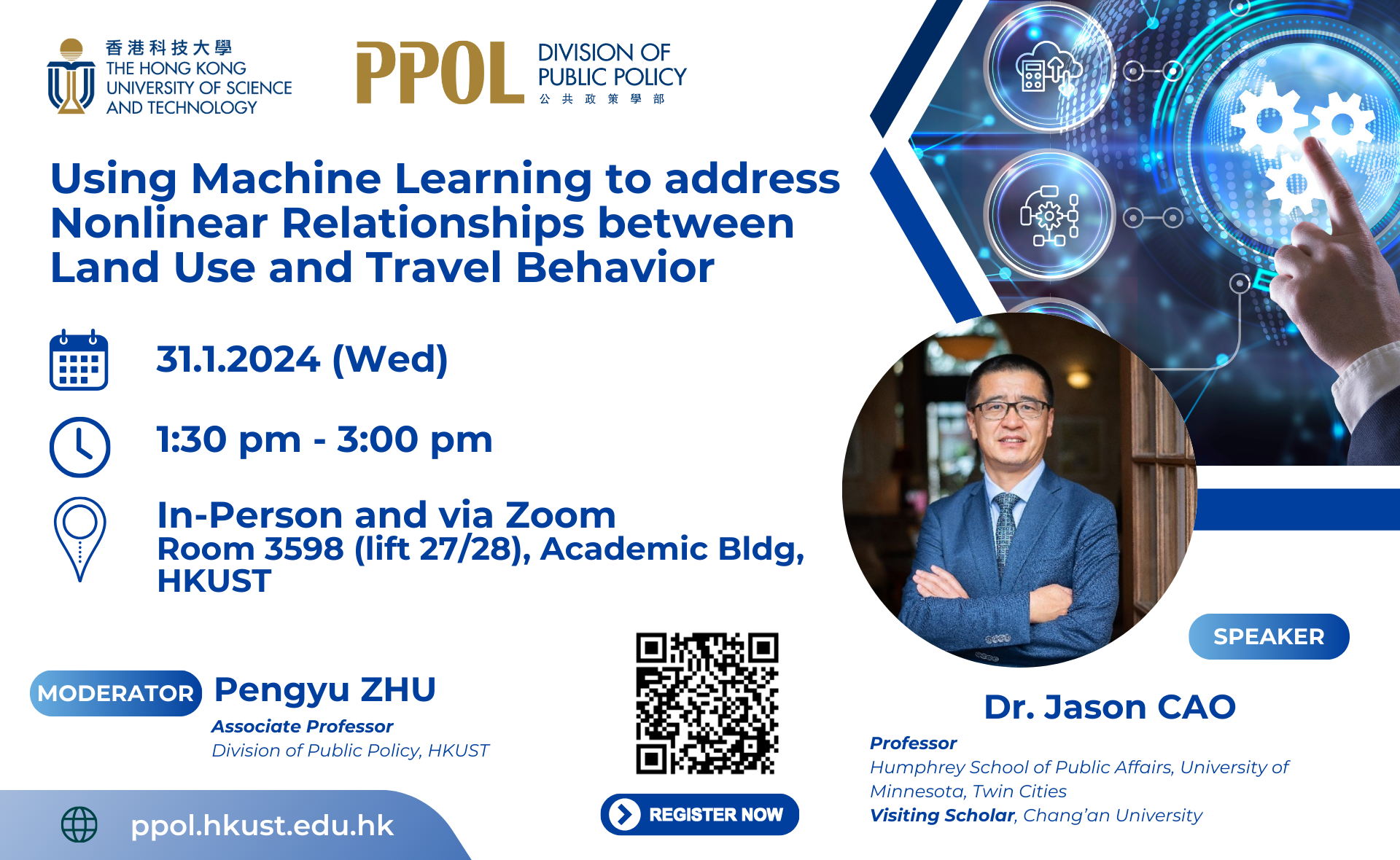
Abstract
Empirical studies often assume a (generalized) linear relationship between variables. However, scholars need to diagnose this assumption before interpreting modeling results. If the true relationship between variables is nonlinear, the linear assumption will lead to a biased estimate of the relationship. This presentation emphasizes the charm of machine learning approaches in address the nonlinear relationships between variables in the context of land use and travel behavior. The case studies show that the applications of machine learning may change the conventional understanding of land use-transportation interactions and inform urban and transportation planners of efficient solutions to address transportation-related challenges. The approaches enable scholars and practitioners to revisit previous questions from an innovative perspective.
Dr. Jason Cao is a professor at the Humphrey School of Public Affairs, University of Minnesota, Twin Cities and a visiting scholar of Chang’an University. He specializes in land use and transportation interaction, the effects of ICT on travel behavior, and planning for quality of life. He has published more than 140 peer-reviewed papers and edited four books. Dr. Cao is internationally well-known for his research on residential self-selection in the relationships between the built environment and travel behavior. He is currently leading the area of machine learning applications in land use and transportation research. Dr. Cao is the Co-Editor-in-Chief of Transportation Research Part D and an associate editor of Transport Policy. Dr. Cao received his degrees from University of California, Davis and Tsinghua University.

Empirical studies often assume a (generalized) linear relationship between variables. However, scholars need to diagnose this assumption before interpreting modeling results. If the true relationship between variables is nonlinear, the linear assumption will lead to a biased estimate of the relationship. This presentation emphasizes the charm of machine learning approaches in address the nonlinear relationships between variables in the context of land use and travel behavior. The case studies show that the applications of machine learning may change the conventional understanding of land use-transportation interactions and inform urban and transportation planners of efficient solutions to address transportation-related challenges. The approaches enable scholars and practitioners to revisit previous questions from an innovative perspective.